
We study learning in brains and machines
ABOUT MY WORK
Brains develop and change following experience. Using a wide variety of neurobiological data from electrophysiology to in-situ hybridization and RNA sequencing, we quantify how plasticity is implemented in neural tissues.
Machines can also learn from examples. We develop algorithms that allow machines to represent complex signals and learn effectively at large scale. We teach our machines to recognize sounds and understand rich visual scenes.
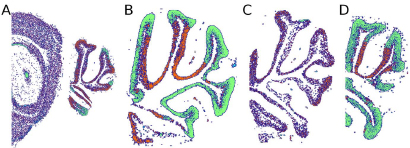
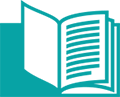
SELECTED PUBLICATIONS
Context-aware Captions from Context-agnostic Supervision
R. Vedantam, S. Bengio, K. Murphy, D. Parikh, G. Chechik | CVPR 2017
Group online adaptive learning
A. Zweig, G. Chechik | J. Machine Learning pp 1-24 2017
Activity motifs reveal principles of timing in transcriptional control of the yeast metabolic network
G. Chechik, E. Oh, O. Rando, J. Weissman, A. Regev and D. Koller | Nature Biotechnology, 26(11) pp 1251-1259. Nov 2008
Auditory abstraction from spectro-temporal features to coding auditory entities
G. Chechik, I Nelken | Proc. Natl. Acad. Science, PNAS, 109(46) 2012
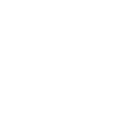
RESEARCH PROJECTS
Learning with rich supervision.
Understanding complex visual scenes.
Reading the brain by decoding precise MEG activity.